Review | Open Access
Development and advanced applications of hepatobiliary tumor organoid models in drug response prediction
Changhua Zeng1, Hongtan Lin1, Yanxiong Liu1, Shen Chen1
1Department of General Surgery, Sanming First Hospital, Sanming City, Fujian Province, China.
Correspondence: Changhua Zeng (Department of General Surgery, Sanming First Hospital, No. 29, Dongxin 1st Road, Liedong Street, Meili District, Sanming City, Fujian Province, China; Email: emerglin@126.com )
Asia-Pacific Journal of Oncology 2024, 5: 16-22. https://doi.org/10.32948/ajo.2024.08.06
Received: 24 Jul 2024 | Accepted: 08 Aug 2024 | Published online: 25 Aug 2024
Key words hepatobiliary tumors, organoid models, drug response prediction, hepatocellular carcinoma, intrahepatic cholangiocarcinoma, gallbladder cancer, three-dimensional culture technology, individualized medicine, drug screening, precision medicine
Organoid models have recently gained popularity in tumor research as one of the advanced in vitro models because of their in vivo like tumor microenvironment and biological properties [6-8]. Organoids are small structures developed from adult stem cells or pluripotent stem cells by 3D culture methods in vitro with self-stemness and multi-lineage differentiation potential [9-11]. These organelloid structures can preserve the histological, molecular, and genetic integrity of the original tumors and reconstruct only limited cellular components of the tumor microenvironment and biological activity, giving an artificial system that is more physiologically relevant for tumor studies [12].
In contrast to the ordinary 2D cell culture and animal models, organoid models have the following benefits. Organoid models are superior to 2D cultures for predicting molecular and biophysical properties of tumor cells and their relationships with neighbors, the extracellular matrix, and changes to the tumor microenvironment. Furthermore, high levels of individualization are possible in organoid models, which creates tissue-specific organoids using a patient’s tumor cell or tissue samples promoting personalized medicine [13-16]. Moreover, organoid cultures are more accessible to develop, cost-effective, and highly efficient for large-scale applications, particularly in drug discovery.
Organoid models are becoming popular for studying hepatobiliary tumors very quickly. These models are used to investigate tumor formation, testing potential drugs and providing opportunities to design individual therapeutic regimens [17, 18]. Applying organoid models, it is possible to quickly assess the cytotoxic response of tumour cells to the drug and determine the drug’s sensitivity and resistance, which allows to develop individual treatment strategies for patients [19]. In addition, organoid models can herein determine how the tumor microenvironment influences drug resistance, analyse pathways by which tumors evade immune recognition, and help identify novel immunotherapies [20]. The clinical treatment of hepatobiliary tumors has multiple difficulties and uncertainties. As a novel platform of research, organoid models bring fresh directions and approaches for the modal understanding and pharmacological treatment of HBTs. Based on the current progress and uses of organoid technology, it is possible to think that these models will be useful in the concept of precision medicine for HB tumors.
Organoid models require the use of three-dimensional (3D) culture techniques [26]. Unlike the conventional two dimensional with flat surface 2D culture system, 3D culture techniques allow cells to be closer to their physiological setting. Under these conditions, cells are capable of perceiving the extracellular space and substrate, and may form tissues with precise three-dimensional organization [27-29]. In addition, 3D cultures enable cell-cell communication and signaling required for preservation of cellular phenotype and function [30]. Organoid models also have highly valuable characteristic such as the self-renewal capability. In certain culture settings and with appropriate signaling control, the stem cells of the organoid models are capable of multiple rounds of symmetric division, so that the organoid models remain stable in the long term [31, 32]. The long-term expansion competency is invaluable not only for the study of stem cells but also for possible applications in regenerative medicine and cell therapy.
The features underscore that organoid models possess specific analytical capabilities in modeling liver pathophysiologic processes [33-36]. Liver is one of the most multifaceted organ of the organism its activity is associated with metabolism, detoxication, immune response etc. Conventional two-dimensional cell cultures lack proper representation of these physiological functions, while the organoids provide a partial representation of liver functions. For instance, liver organoids may exhibit hepatic enzymes and transporter proteins, and perform xenobiotic and drug metabolism and bile production [37]. Moreover, liver organoids are very helpful in understanding the development and progression of liver diseases, they are important models in explaining the molecular basis of liver diseases [38]. Nevertheless, there are still challenges when using liver organoid models for the simulation of liver pathophysiology despite the benefits. For instance, the problem of recreating the necessary conditions within the niche, such as blood and oxygen circulation, and interactions with immune cells in a liver microenvironment still persists today [39-41]. Furthermore, several questions remain unanswered in the field of organoid development: the enhancement of stability and reproducibility of organoids; and the use of organoid models for large-scale drug screening, toxicity tests [39]. Organoid models are emerging as a promising in vitro approach, particularly for simulating complex liver pathophysiological mechanisms. With ongoing advancements and refinements in this technology, organoid models are poised to provide increasingly accurate and efficient methodologies for liver disease research and treatment [40]. As we continue to explore and apply organoids in the context of hepatobiliary tumor investigations and therapeutics, these models are expected to significantly enhance our capabilities, ultimately offering patients more effective and personalized treatment options [41].
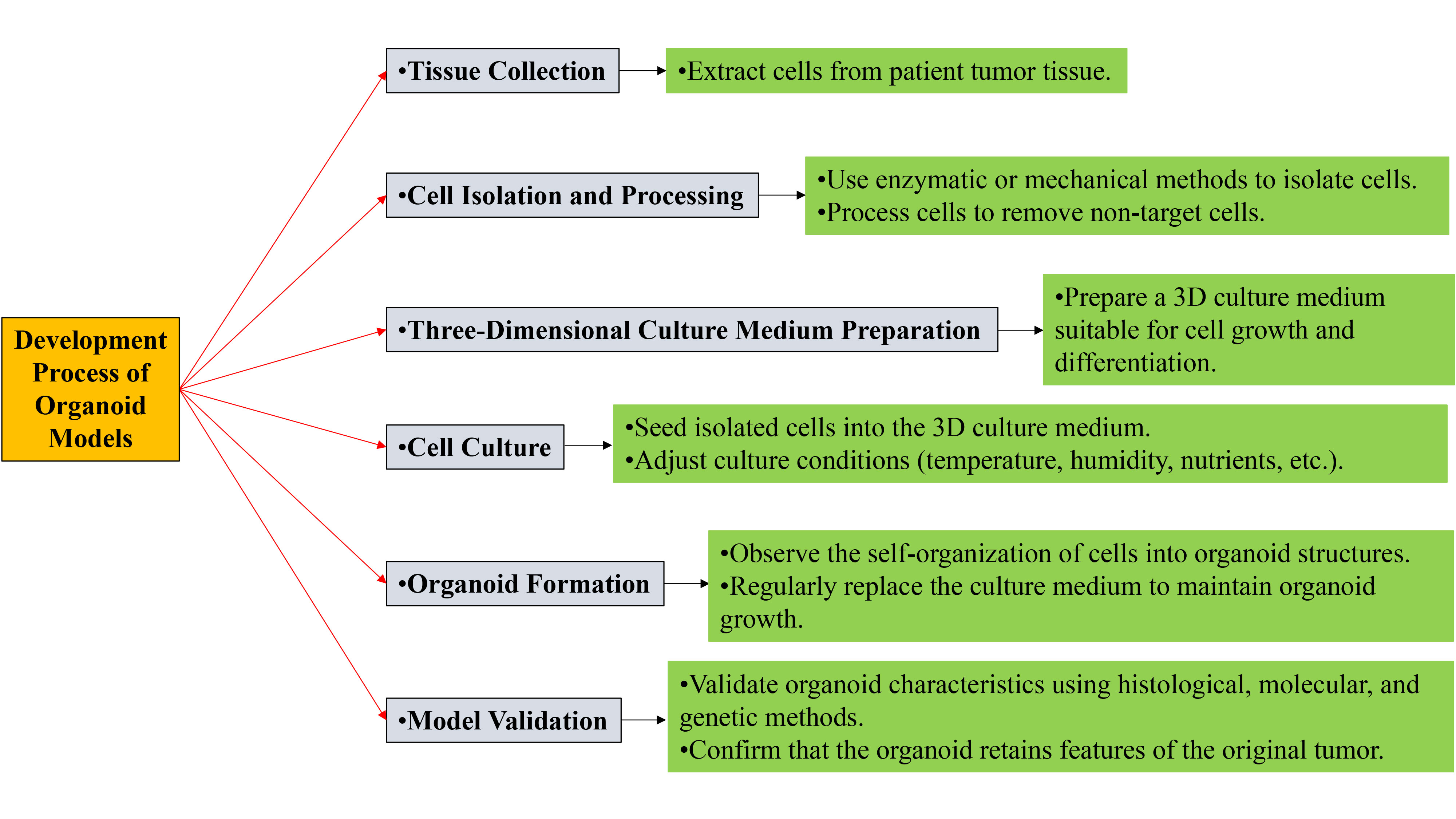
In the context of disease models, hepatobiliary tumor organoid models are widely used to analyze tumor formation, progression, metastasis and chemoresistance. Tumor cells can be implanted in a system which is nearest to vivo, and researchers can see cell growth, division and behavior. Also, organsoid models allow understanding of cell interactions within the tumor niche and how tumors can escape immune recognition and destruction [47].
The strength in utilizing organoid models is in maintaining the phenotypic profile of primary tumors. Compared to 2D cell culture and animal models organoids recapitulate tumours’ 3D architecture and cell-to-cell communication [48, 49]. Thus, this highly mimetic feature makes organoid models perfect for investigating tumor heterogeneity and the effect of the milieu. Also, as organoid culture models employed a single-cell approach, they also enable personalized medicine because of individual differences. Organoids generated from a patient’s tumor cells imply that the development of treatment plan tailor to the patient’s case is made possible [48, 49].
Specifically worth mentioning are Patient-Derived Hepatobiliary Organoids (PDHO). PDHO models can apply at high levels for the prediction of drug sensitivity of hepatobiliary tumors [50-52]. Thanks to the usage of different drugs for tumor cells within the frameworks of PDHO models, investigators can predict the effectiveness of drugs and their toxic effects in the treatment of patients. Furthermore, the PDHO topological models are useful for the HTS in drug discovery [53-55]. By using such models, it is easy for the researchers to discover compounds with antitumor activity since the effects of several compounds can be tested on the models at once, hence shortening the process of identifying new drugs. However, the development and implementation of PDHO models are not problem-free either. For instance, the culture conditions used for organoids must be made to assume the various types of tumors in order to guarantee model stability and replicability [53, 54]. Furthermore, due to tumorigenesis heterogeneity, the specific organoid model may not capture all aspects of the tumor behavior, which requires multiple vehicle models towards a comprehensive understanding of tumorigenesis. However, there are still many points to improve, and thus the application prospects of PDHO models in hepatobiliary tumor research and treatment are still very brilliant [55].
As technology and knowledge of tumour biology progress, hepatobiliary tumor organoid models will be progressively important in tumour research and therapy. They include essential knowledge of the tumor notion and contribute to the establishment of individualized and effective treatment approaches to promote benefits in patients in terms of quality of life.
Whole-genome sequencing (WGS) is playing an increasingly important role in cancer research. Through WGS, researchers can gain a comprehensive understanding of the genomic information of both tumor and normal cells, providing strong support for the diagnosis and treatment of cancer [56]. For example, in a study, WGS was used to identify germline variants associated with the susceptibility to colorectal cancer (CRC), such as mutations in the POLE and POLD1 genes, which are related to a high penetrance of CRC [57]. Additionally, WGS has been used to identify other genes associated with cancer susceptibility, such as TP53 and ATM gene mutations [58].
RNA sequencing (RNA-seq) technology is crucial in analyzing the transcriptome of tumor cells. It can reveal the adaptability of tumor cells to changes in their microenvironment and therapeutic interventions, and by identifying differential gene expression profiles, it provides a basis for the molecular classification and prognostic assessment of tumors [59]. For instance, in a study on oral cancer, RNA-Seq was used to explore the genetic basis related to the disease [60]. Moreover, RNA-seq has also been used to analyze miRNAs and other non-coding small RNAs in formalin-fixed paraffin-embedded (FFPE) tumor samples, which may contain important information about the pathogenesis and outcomes of cancer [61].
Mass spectrometry (MS) technology provides important information at the proteomic level for tumor research. It can quantitatively profile the expression and post-translational modifications of proteins in tumor cells, revealing the metabolic characteristics of tumor cells and the dynamic activities of drugs [62]. For example, in one study, MS technology was used to analyze the glycoforms of prostate-specific antigen (PSA) to improve the accuracy of prostate cancer diagnosis [63]. Additionally, MS technology has been used to explore biomarkers in plasma, providing potential targets for the early diagnosis and treatment of cancer [64].
The development of single-cell RNA sequencing (scRNA-seq) technology has provided a new perspective for the study of tumor heterogeneity. Through single-cell resolution transcriptome analysis, researchers can reveal the unique characteristics and states of different cellular subsets within tumors, which is crucial for understanding tumor development, metastasis, and therapeutic resistance [65]. For example, in a study, scRNA-seq was used to analyze the tumor microenvironment of esophageal squamous cell carcinoma (ESCC), revealing the heterogeneity of lymphoid and myeloid cell lineages, and identifying a tumor-specific subset of CST1+ myofibroblasts with significant prognostic value and potential biological significance [66]. Furthermore, the CancerSCEM database developed by the Beijing Institute of Genomics, Chinese Academy of Sciences, integrates single-cell data from various types of cancers, providing rich resources for the study of the cancer microenvironment [67].
Combined with these multi-omics technologies, it is possible to systematically decipher the developmental processes of hepatobiliary tumors on the molecular level. For example, WGS and RNA-seq can jointly allow for the discovery of mutations that exist in the tumor cells as well as examination of the effects of such mutations on gene expression and the behavior of the tumor [68]. The integration of MS and scRNA-seq reveals the proteins and single cell heterogeneity within the tumor cells that can be used as leads to new treatment interventions. Moreover, the use of multi-omics in organoid models allow for an assessment of drug response and an orientation toward individualized therapy. A quantitative analysis of the signaling response of tumors to various drugs enables investigators to discover the biomarkers of drugs’ efficacy and toxicity. They harbour the patient’s clinical data along with multi-omics data of desired organoid models for treatment planning leading to enhancement in treatment efficacies [69].
The applicability of the organoid models in drug scrutiny and assessment of effectiveness is clear. With organoid models, researchers are able to work with a tumor cells in order to evaluate the effectiveness of the drugs and, at the same time, measure potential toxicity of the drug. This damage comprises the immediate lethal actions upon tumor cells and the effects on the tumor milieu, including angiogenesis and immune modulation [72]. More significantly, organoid models can model the tumor heterogeneity, meaning that the model can show the response of the multiple subpopulations of tumor cells to drugs and therefore provide a richer information set for drug testing.
The potential of intensively investigated in vitro screening platforms based on organoids with respect to neoantigen peptide activity has also emerged in the field of personalised immunotherapies. The tumour neoepitopes that are generated from tumour associated nonsynonymous mutations can activate the immune response against tumour cells [73, 74]. By doing so, researchers can pinpoint positive, or neoantigen, peptides that can activate the immune system with the use of organoid models. Thus, this method can extend and improve immunotherapy and decrease negative outcomes. Therefore, methods for predicting drug-target interactions, including the wSDTNBI method, also have an important role in drug discovery. The wSDTNBI method, an acronym for Weighted Short-Driven Time New Bariccentric Index, employs a sophisticated network-based strategy to forecast the efficacy of drugs in engaging their molecular targets [73]. By incorporating the weighted influences and temporal dynamics of drug-target interactions, this method extends its utility beyond mere prediction, offering a nuanced assessment of the interaction's intensity and specificity [74]. This level of detail is crucial for optimizing drug design, as it allows researchers to tailor compounds to more effectively interact with their intended targets. The wSDTNBI method thus serves as a powerful tool in the arsenal of drug discovery, enabling the development of more precise and potent therapeutics [75].
Indeed, the wSDTNBI method has potential application in the prediction of the activity of several drugs against diverse targets [75]. Originally, by employing this approach, researchers can easily-screen compounds which might possess activity, saving time and increasing the process of drug identification. In addition, no matter target or negative samples, the wSDTNBI method does not necessitate the 3D structure information, so that it can be used broadly in the drug discovery field.
Of course, both, organoid models, and drug-target interaction prediction methods have many opportunities in predicting drug responses. Ex situ culture and maintenance of organoids require intricate procedures and conditions in their formation. Additionally, the substantial interpatient variability of tumor cells diminishes the model’s representative and reproducible nature [76, 77]. Furthermore, even though several computer-aided drug-target interaction prediction methods can give relatively fast predictions, the prediction quality and necessity of further optimization remain questionable.
Altogether, organoid models and drug-target interaction prediction approaches have a great potential for enhancing the prediction of drugs’ effect. As technology ceases not to progress and these methods continually get optimized, more accurate and individualized approaches of cancer will be developed hence coming up with better results by increasing the survival rates of the patient. In future, through integration of multi-disciplinary fields and technological advancement, many higher progressive results are foreseen in treating cancer.
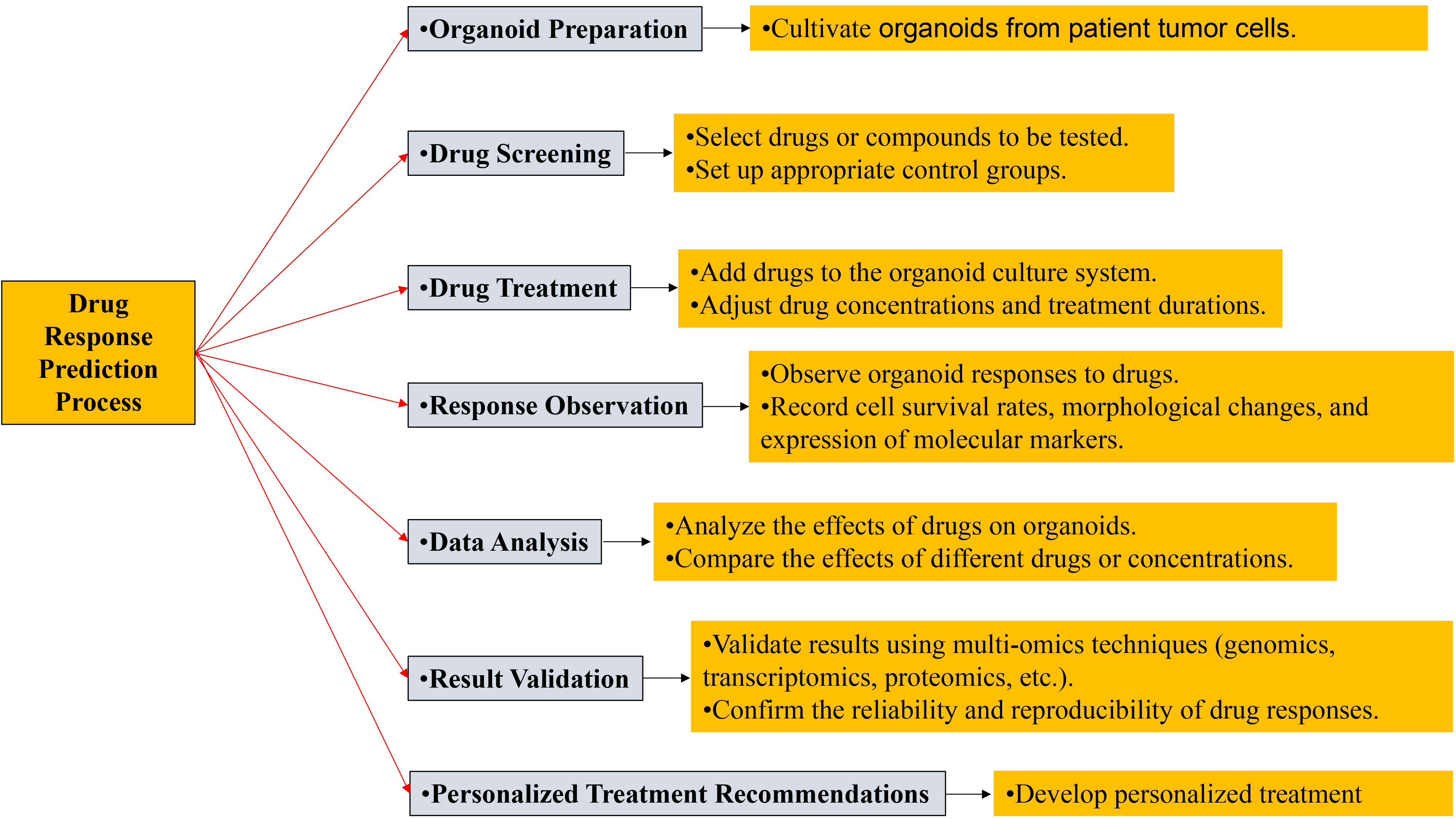
Despite their potential, organoid models present certain challenges when translated to clinical use. A primary concern is the complexity of their internal structure, which necessitates a delicate balance between tumor cell characteristics and microenvironmental variations. Replicating the intricacies of the natural tumor milieu within these models is a complex endeavor. Furthermore, the significant inter-patient heterogeneity observed within the same cancer type adds another layer of complexity; organoid models must capture the unique features of each patient's tumor to be clinically relevant.
To surmount these challenges, a variety of strategies have been implemented to refine culture techniques and integrate multi-omics data [79]. By optimizing the composition of culture media and conditions, organoid models can achieve greater stability and fidelity. Additionally, the integration of diverse omics layers, including genomics, transcriptomics, proteomics, and metabolomics, deepens our understanding of the molecular underpinnings of tumors and bolsters the predictive power of these models. Looking ahead, future research is poised to enhance our grasp of the evolution of organoid models and drug response prediction technologies, especially within the field of hepatobiliary tumor research [79]. The synergy between cutting-edge technological advancements and a profound comprehension of tumor biology is anticipated to elevate the capacity of these models to emulate the tumor microenvironment and biological behaviors. This, in turn, will bolster drug screening and pave the way for more tailored treatment approaches. Continued progress in this domain will likely stem from interdisciplinary collaboration and technological innovation, with the convergence of bioinformatics, systems biology, and clinical medicine potentially yielding more robust and reliable models and predictive methodologies.
Data and materials are available on request from the authors.
Ethical policy
Not applicable.
Author contributions
CHZ and HTL conceptualized, designed, conducted research, and wrote the first draft; YXL contributed to the revision and figure production; SC provided supervision and revision of the draft.
Competing interests
The authors have no conflicts of interest regarding the publication of this paper.
Funding
None.
- Argemi J, Ponz-Sarvise M, Sangro B: Immunotherapies for hepatocellular carcinoma and intrahepatic cholangiocarcinoma: current and developing strategies. Adv Cancer Res 2022, 156: 367-413.
- Hormati A, Hajrezaei Z, Jazi K, Aslani Kolur Z, Rezvan S, Ahmadpour S: Gastrointestinal and pancratohepatobiliary cancers: a comprehensive review on epidemiology and risk factors worldwide. Middle East J Dig Dis 2022, 14(1): 5-23.
- Liao X, Zhang D: The 8th edition American Joint Committee on cancer staging for hepato-pancreato-biliary cancer: a review and update. Arch Pathol Lab Med 2021, 145(5): 543-553.
- Marin JJG, Prete MG, Lamarca A, Tavolari S, Landa-Magdalena A, Brandi G, Segatto O, Vogel A, Macias RIR, Rodrigues PM, et al: Current and novel therapeutic opportunities for systemic therapy in biliary cancer. Br J Cancer 2020, 123(7): 1047-1059.
- Lohitesh K, Chowdhury R, Mukherjee S: Resistance a major hindrance to chemotherapy in hepatocellular carcinoma: an insight. Cancer Cell Int 2018, 18: 44.
- Mao S, Pang Y, Liu T, Shao Y, He J, Yang H, Mao Y, Sun W: Bioprinting of in vitro tumor models for personalized cancer treatment: a review. Biofabrication 2020, 12(4): 042001.
- Clevers H, Tuveson DA: Organoid models for cancer research. Annu Rev Cancer Biol 2019, 3(1): 223-234.
- Manduca N, Maccafeo E, De Maria R, Sistigu A, Musella M: 3D cancer models: One step closer to in vitro human studies. Front Immunol 2023, 14: 1175503.
- Jin H, Xue Z, Liu J, Ma B, Yang J, Lei L: Advancing Organoid Engineering for Tissue Regeneration and Biofunctional Reconstruction. Biomater Res 2024, 28: 0016.
- Long HY, Qian ZP, Lan Q, Xu YJ, Da JJ, Yu FX, Zha Y: Human pluripotent stem cell-derived kidney organoids: Current progress and challenges. World J Stem Cells 2024, 16(2): 114-125.
- Wu H, Wang G, Shang Y, Liu N, Zhen Y, Chen L, Li H, An Y: Organoids and their research progress in plastic and reconstructive surgery. Aesthet Plast Surg 2023, 47(2): 880-891.
- Tan ML, Ling L, Fischbach C: Engineering strategies to capture the biological and biophysical tumor microenvironment in vitro. Adv Drug Deliv Rev 2021, 176: 113852.
- Kaushik G, Ponnusamy MP, Batra SK: Concise review: current status of three-dimensional organoids as preclinical models. Stem Cells 2018, 36(9): 1329-1340.
- Wu X, Su J, Wei J, Jiang N, Ge X: Recent advances in three‐dimensional stem cell culture systems and applications. Stem Cells Int 2021, 2021: 9477332.
- Lee SY, Koo IS, Hwang HJ, Lee DW: In Vitro three-dimensional (3D) cell culture tools for spheroid and organoid models. SLAS Discov 2023, 28(4): 119-137.
- Gunti S, Hoke ATK, Vu KP, London NR Jr: Organoid and spheroid tumor models: techniques and applications. Cancers 2021, 13(4): 874.
- Dienstmann R, De Dosso S, Felip E, Tabernero J: Drug development to overcome resistance to EGFR inhibitors in lung and colorectal cancer. Mol Oncol 2012, 6(1): 15-26.
- Dermawan JK, Rubin BP: Molecular pathogenesis of gastrointestinal stromal tumor: a paradigm for personalized medicine. Annu Rev Pathol 2022, 17(1): 323-344.
- Verduin M, Hoeben A, De Ruysscher D, Vooijs M: Patient-derived cancer organoids as predictors of treatment response. Front Oncol 2021, 11: 641980.
- Rahman MM, Wells G, Rantala JK, Helleday T, Muthana M, Danson SJ: In-vitro assays for immuno-oncology drug efficacy assessment and screening for personalized cancer therapy: scopes and challenges. Expert Rev Clin Immunol 2024, 20(8): 821-838.
- Prasad M, Kumar R, Buragohain L, Kumari A, Ghosh M: Organoid technology: A reliable developmental biology tool for organ-specific nanotoxicity evaluation. Front Cell Dev Biol 2021, 9: 696668.
- Sadeghzade S, Hosseini Hooshiar M, Akbari H, Mahdi Tajer MH, Kaki Sahneh K, Yousefpour Ziaei S, Jalali F, Akouchakian E: Recent advances in Organ-on-a-Chip models: How precision engineering integrates cutting edge technologies in fabrication and characterization. Appl Mater Today 2024, 38: 102231.
- Wang Y, Qin J: Advances in human organoids-on-chips in biomedical research. Life Med 2023, 2(1): lnad007.
- Jackson EL, Lu H: Three-dimensional models for studying development and disease: moving on from organisms to organs-on-a-chip and organoids. Integr Biol 2016, 8(6): 672-683.
- Luo Z, Zhou X, Mandal K, He N, Wennerberg W, Qu M, Jiang X, Sun W, Khademhosseini A: Reconstructing the tumor architecture into organoids. Adv Drug Deliv Rev 2021, 176: 113839.
- Ren X, Chen W, Yang Q, Li X, Xu L: Patient‐derived cancer organoids for drug screening: basic technology and clinical application. J Gastroenterol Hepatol 2022, 37(8): 1446-1454.
- Knight E, Przyborski S: Advances in 3D cell culture technologies enabling tissue‐like structures to be created in vitro. J Anat 2015, 227(6): 746-756.
- Habanjar O, Diab-Assaf M, Caldefie-Chezet F, Delort L: 3D cell culture systems: tumor application, advantages, and disadvantages. Int J Mol Sci 2021, 22(22): 12200.
- Mirbagheri M, Adibnia V, R. Hughes B, D. Waldman S, Banquy X, Kun Hwang D: Advanced cell culture platforms: a growing quest for emulating natural tissues. Mater Horiz 2019, 6(1): 45-71.
- Ravi M, Ramesh A, Pattabhi A: Contributions of 3D cell cultures for cancer research. J Cell Physiol 2017, 232(10): 2679-2697.
- Wu X, Su J, Wei J, Jiang N, Ge X: Recent advances in three-dimensional stem cell culture systems and applications. Stem Cells Int 2021, 2021: 9477332.
- Tang XY, Wu S, Wang D, Chu C, Hong Y, Tao M, Hu H, Xu M, Guo X, Liu Y: Human organoids in basic research and clinical applications. Signal Transduct Target Ther 2022, 7(1): 168.
- Lee SW, Jung DJ, Jeong GS: Gaining new biological and therapeutic applications into the liver with 3D in vitro liver models. Tissue Eng Regen Med 2020, 17(6): 731-745.
- Akbari S, Arslan N, Senturk S, Erdal E: Next-generation liver medicine using organoid models. Front Cell Dev Biol 2019, 7: 345.
- Fiorotto R, Amenduni M, Mariotti V, Fabris L, Spirli C, Strazzabosco M: Liver diseases in the dish: iPSC and organoids as a new approach to modeling liver diseases. Biochim Biophys Acta Mol Basis Dis 2019, 1865(5): 920-928.
- Bao Y-l, Wang L, Pan H-t, Zhang T-r, Chen Y-h, Xu S-j, Mao X-l, Li S-w: Animal and organoid models of liver fibrosis. Front Physiol 2021, 12: 666138.
- Mitaka T, Ooe H: Characterization of hepatic-organoid cultures. Drug Metab Rev 2010, 42(3): 472-481.
- Yuan B, Zhao X, Wang X, Liu E, Liu C, Zong Y, Jiang Y, Hou M, Chen Y, Chen L, et al: Patient‐derived organoids for personalized gallbladder cancer modelling and drug screening. Clin Transl Med 2022, 12(1): e678.
- Clark AM, Ma B, Taylor DL, Griffith L, Wells A: Liver metastases: Microenvironments and ex-vivo models. Exp Biol Med 2016, 241(15): 1639-1652.
- Bao YL, Wang L, Pan HT, Zhang TR, Chen YH, Xu SJ, Mao XL, Li SW: Engineering liver microtissues for disease modeling and regenerative medicine. Adv Funct Mater 2020, 30(44): 1909553.
- Huang D, Gibeley SB, Xu C, Xiao Y, Celik O, Ginsberg HN, Leong KW: 3D liver models on a microplatform: well-defined culture, engineering of liver tissue and liver-on-a-chip. Lab Chip 2015, 15(19): 3822-3837.
- H Fang, H Xu, J Yu, H Cao, L Li: Human Hepatobiliary Organoids: Recent Advances in Drug Toxicity Verification and Drug Screening. Biomolecules 2024, 14(7): 794.
- Hare JI, Lammers T, Ashford MB, Puri S, Storm G, Barry ST: Challenges and strategies in anti-cancer nanomedicine development: An industry perspective. Adv Drug Deliv Rev 2017, 108: 25-38.
- Moffat JG, Vincent F, Lee JA, Eder J, Prunotto M: Opportunities and challenges in phenotypic drug discovery: an industry perspective. Nat Rev Drug Discov 2017, 16(8): 531-543.
- Zhou BB, Zhang H, Damelin M, Geles KG, Grindley JC, Dirks PB: Tumour-initiating cells: challenges and opportunities for anticancer drug discovery. Nat Rev Drug Discov 2009, 8(10): 806-823.
- Castro F, Leite Pereira C, Helena Macedo M, Almeida A, José Silveira M, Dias S, Patrícia Cardoso A, José Oliveira M, Sarmento B: Advances on colorectal cancer 3D models: The needed translational technology for nanomedicine screening. Adv Drug Deliv Rev 2021, 175: 113824.
- Zhang J, Tavakoli H, Ma L, Li X, Han L, Li X: Immunotherapy discovery on tumor organoid-on-a-chip platforms that recapitulate the tumor microenvironment. Adv Drug Deliv Rev 2022, 187: 114365.
- Imparato G, Urciuolo F, Netti PA: In vitro three-dimensional models in cancer research: a review. Int Mater Rev 2015, 60(6): 297-311.
- Van Zundert I, Fortuni B, Rocha S: From 2D to 3D cancer cell models—the enigmas of drug delivery research. Nanomaterials 2020, 10(11): 2236.
- Zhu Y, Tang S, Yuan Q, Fu J, He J, Liu Z, Zhao X, Li Y, Zhao Y, Zhang Y, et al: Integrated characterization of hepatobiliary tumor organoids provides a potential landscape of pharmacogenomic interactions. Cell Rep Med 2024, 5(2): 101375.
- Wang W , Ma L , Xing Z , Yuan T, Bao J, Zhu Y, Zhao X, Zhao Y, Zong Y, Zhang Y, et al: Tumor-Specific CircRNA-Derived Antigen Peptide Identification for Hepatobiliary Tumors. Engineering 2023, 22: 159-170.
- Zhu Y, Tang S, Yuan Q, Fu J, He J, Liu Z, Zhao X, Li Y, Zhao Y, Zhang Y, et al: Integrated characterization of hepatobiliary tumor organoids provides a potential landscape of pharmacogenomic interactions. Cell Rep Med 2024, 5(2): 101375.
- Saito Y: Establishment of an organoid bank of biliary tract and pancreatic cancers and its application for personalized therapy and future treatment. J Gastroenterol Hepatol 2019, 34(11): 1906-1910.
- Liu XM, Chapman GB, Wang H, Durante W: Adenovirus-mediated heme oxygenase-1 gene expression stimulates apoptosis in vascular smooth muscle cells. Circulation 2002, 105(1): 79-84.
- Nageswara Rao AA, Warad DM, Weaver AL, Schleck CD, Rodriguez V: Cross-cultural medical care training and education: a national survey of pediatric hematology/oncology fellows-in-training and fellowship program directors. J Cancer Educ 2019, 34(3): 478-487.
- Hou YC, Neidich JA, Duncavage EJ, Spencer DH, Schroeder MC: Clinical whole‐genome sequencing in cancer diagnosis. Hum Mutat 2022, 43(11): 1519-1530.
- Belhadj S, Terradas M, Munoz‐Torres PM, Aiza G, Navarro M, Capella G, Valle L: Candidate genes for hereditary colorectal cancer: Mutational screening and systematic review. Hum Mutat 2020, 41(9): 1563-1576.
- Giunta S: Decoding human cancer with whole genome sequencing: a review of PCAWG Project studies published in February 2020. Cancer Metastasis Rev 2021, 40(3): 909-924.
- Qian Y, Daza J, Itzel T, Betge J, Zhan T, Marmé F, Teufel A: Prognostic cancer gene expression signatures: current status and challenges. Cells 2021, 10(3): 648.
- Wang H, Deng Y, Peng S, Yan L, Xu H, Wang Q, Shen Z: RNA-Seq based transcriptome analysis in oral lichen planus. Hereditas 2021, 158(1): 39.
- Majed SO, Mustafa SA: MACE-Seq-based coding RNA and TrueQuant-based small RNA profile in breast cancer: tumor-suppressive miRNA-1275 identified as a novel marker. BMC cancer 2021, 21(1): 473.
- Li W, Li F, Zhang X, Lin HK, Xu C: Insights into the post-translational modification and its emerging role in shaping the tumor microenvironment. Signal Transduct Targeted Ther 2021, 6(1): 422.
- Gabriele C, Prestagiacomo LE, Cuda G, Gaspari M: Mass spectrometry-based glycoproteomics and prostate cancer. Int J Mol Sci 2021, 22(10): 5222.
- Macklin A, Khan S, Kislinger T: Recent advances in mass spectrometry based clinical proteomics: applications to cancer research. Clin Proteomics 2020, 17(1): 17.
- Han Y, Wang D, Peng L, Huang T, He X, Wang J, Ou C: Single-cell sequencing: a promising approach for uncovering the mechanisms of tumor metastasis. J Hematol & Oncol 2022, 15(1): 59.
- Dinh HQ, Pan F, Wang G, Huang QF, Olingy CE, Wu ZY, Li EM: Integrated single-cell transcriptome analysis reveals heterogeneity of esophageal squamous cell carcinoma microenvironment. Nat Commun 2021, 12(1): 7335.
- Du WQ, Zhu ZM, Jiang X, Kang MJ, Pei DS: COPS6 promotes tumor progression and reduces CD8+ T cell infiltration by repressing IL-6 production to facilitate tumor immune evasion in breast cancer. Acta Pharmacol Sin 2023, 44(9): 1890-1905.
- de Joode K, van de Geer WS, van Leenders GJLH, Hamberg P, Westgeest HM, Beeker A, Oosting SF, van Rooijen JM, Beerepoot LV, Labots M, et al: The genomic and transcriptomic landscape of advanced renal cell cancer for individualized treatment strategies. Sci Rep 2023, 13(1): 10720.
- Feng F, Shen B, Mou X, Li Y, Li H: Large-scale pharmacogenomic studies and drug response prediction for personalized cancer medicine. J Genet Genomics 2021, 48(7): 540-551.
- Lee RY, Wu Y, Goh D, Tan V, Ng CW, Lim JCT, Lau MC, Yeong JPS: Application of artificial intelligence to in vitro tumor modeling and characterization of the tumor microenvironment. Adv Healthc Mater 2023, 12(14): 2202457.
- Kuenzi BM, Park J, Fong SH, Sanchez KS, Lee J, Kreisberg JF, Ma J, Ideker T: Predicting drug response and synergy using a deep learning model of human cancer cells. Cancer Cell 2020, 38(5): 672-684.e6.
- Jiang X, Wang J, Deng X, Xiong F, Zhang S, Gong Z, Li X, Cao K, Deng H, He Y, et al: The role of microenvironment in tumor angiogenesis. J Exp Clin Cancer Res 2020, 39(1): 204.
- Fang X, Guo Z, Liang J, Wen J, Liu Y, Guan X, Li H: Neoantigens and their potential applications in tumor immunotherapy. Oncol Lett 2022, 23(3): 1-9.
- Benvenuto M, Focaccetti C, Izzi V, Masuelli L, Modesti A, Bei R: Tumor antigens heterogeneity and immune response-targeting neoantigens in breast cancer. Semin Cancer Biol 2021, 72: 65-75.
- Amador CA, Barrientos V, Peña J, Herrada AA, González M, Valdés S, Carrasco L, Alzamora R, Figueroa F, Kalergis AM, et al: Spironolactone decreases DOCA–salt–induced organ damage by blocking the activation of T helper 17 and the downregulation of regulatory T lymphocytes. Hypertension 2014, 63(4): 797-803.
- LeSavage BL, Suhar RA, Broguiere N, Lutolf MP, Heilshorn SC: Next-generation cancer organoids. Nat Mater 2022, 21(2): 143-159.
- Zhao Z, Chen X, Dowbaj AM, Sljukic A, Bratlie K, Lin L, Fong ELS, Balachander GM, Chen Z, Soragni A, et al: Organoids. Nat Rev Methods Primers 2022, 2(1): 94.
- Salemme V, Centonze G, Avalle L, Natalini D, Piccolantonio A, Arina P, Morellato A, Ala U, Taverna D, Turco E, et al: The role of tumor microenvironment in drug resistance: emerging technologies to unravel breast cancer heterogeneity. Front Oncol 2023, 13: 1170264.
- Graw S, Chappell K, Washam CL, Gies A, Bird J, Robeson MS 2nd, Byrum SD: Multi-omics data integration considerations and study design for biological systems and disease. Mol Omics 2021, 17(2): 170-185.
Asia-Pacific Journal of Oncology
print ISSN: 2708-7980, online ISSN: 2708-7999
Copyright © Asia Pac J Oncol. This work is licensed under a Creative Commons Attribution-NonCommercial-No Derivatives 4.0 International (CC BY-NC-ND 4.0) License.