Review | Open Access
Research on single-cell transcriptomics in hepatocellular carcinoma
Nauman Shabbir1
1Department of Medicine, Mohtarma Benazir Bhutto Shaheed Medical College, Mirpur, AJK.
Correspondence: Nauman Shabbir (Department of Medicine, Mohtarma Benazir Bhutto Shaheed Medical College, Mirpur, AJK; Email: drnaumanshabbir@gmail.com).
Asia-Pacific Journal of Oncology 2024, 5: 32-38. https://doi.org/10.32948/ajo.2024.08.25
Received: 10 Aug 2024 | Accepted: 30 Aug 2024 | Published online: 07 Sep 2024
Key words hepatocellular carcinoma, single-cell RNA sequencing, tumor microenvironment, immunotherapy, tumor heterogeneity
In the realm of biomedical research, the emergence of single-cell RNA sequencing (scRNA-seq) technology opens novel research opportunities. This technology enables gene expression analysis at the resolution of individual cells, providing an unprecedented new perspective on cellular heterogeneity and complex biological processes. Introduced in 2009, scRNA-seq has evolved with advancements in sequencing technologies and cost reductions. It has become indispensable for studying cellular states, identifying new cell subpopulations, and elucidating disease mechanisms [8]. Compared to traditional bulk cell sequencing (Bulk-seq), scRNA-seq can characterize the instantaneous state of each cell type within each sample, identify intracellular regulatory networks, and understand cell-to-cell communication [9].
The heterogeneity of HCC is observed between tumors in different patients but even within the tumors of the same patient. In this context, applying scRNA-seq technology in HCC research shows great potential. Analyzing gene expression in tumor cells and the surrounding microenvironment at the single-cell level, the scRNA-seq reveals the heterogeneity of HCC, thereby identifying key driving factors in tumor progression and discovering new therapeutic targets. scRNA-seq also provides a new perspective for analyzing TME, enabling the identification of different cell types, including tumor cells, immune cells, and other supporting cells (i.e., stromal cells) and their interactions. This insight offers clues for developing new immunotherapy strategies. The continuous advancement and application of this technology are expected to deepen our understanding of the molecular mechanisms underlying the development of HCC and other diseases, thereby providing a theoretical basis for establishing more effective personalized treatment plans.
Tissue blocks undergo enzymatic digestion and viability assessment to produce high-quality single-cell suspensions. Subsequent library preparation and sequencing rely on "single-cell isolation techniques", such as flow cytometry sorting, laser capture microdissection, limiting dilution, manual cell picking, and droplet-based microfluidic systems [10]. Common scRNA-seq sequencing methods include Smart-seq2, CEL-seq2, Drop-seq, and 10×Chromium. The Smart-seq2 and CEL-seq2 are low-throughput sequencing methods based on microplate technology, while Drop-seq and 10×Chromium are high-throughput methods that use microfluidic systems to create "oil-in-water" droplets for cell isolation [11]. Currently, the predominant commercial technology in use is 10×Chromium by 10×Genomics [12]. This technology captures and encapsulates single cells in Gel Bead-In-Emulsions (GEMs), integrating a barcode for cell identification, an enzyme mix, and oil droplets. Subsequently, the cells are lysed to release mRNA, which comes into contact with reverse transcriptase, poly dT reverse transcription primers, and dNTP substrates to form cDNA with Unique Molecular Identifier (UMI) information. Chemical methods fragment the cDNA into approximately 200-300 bp pieces. Subsequently, the fragments undergo end repair and poly(A) tail addition for cDNA fragment selection. P7 adapters are ligated to the Read2 sequencing primers, and sample indexes are introduced through PCR amplification. Fragment selection is performed to construct a cDNA library containing P5 and P7 adapters. The library structure is shown in Figure 1. Quality control is performed after the library is completed, followed by Illumina sequencers sequencing. After sequencing is completed, data analysis is conducted. The overall workflow is illustrated in Figure 2.
The basic workflow for data analysis
The key steps determining the accuracy and reliability of the final results in 10×Chromium scRNA-seq data include data preprocessing, normalization and batch effect correction, dimensionality reduction, clustering, and subpopulation annotation. The workflow begins with (1) data preprocessing, which includes quality control, genome alignment, and UMI processing using tools such as Cell Ranger, UMI-tools, and zUMIs. The goal is to use tools such as Cell Ranger, UMI-tools, and zUMIs to process the raw FASTQ files. This involves filtering out low-quality reads, performing genome alignment, and handling UMIs to identify and eliminate PCR-generated duplicates. This approach ensures data quality and usability, creating a cell-gene expression matrix. (2) Normalization and Batch Effect Correction: Utilize tools such as Seurat [13] and Harmony [14] to perform data normalization and batch effect correction on scRNA-seq data. This step is fundamental to eliminating technical variation and batch-specific biases, enhancing data integration quality, and improving data interpretation accuracy. (3) Dimensionality Reduction, Clustering, and Subpopulation Annotation: Use algorithms such as t-SNE and UMAP to cluster cells and perform biological annotation of clusters based on gene expression patterns. This step is crucial for understanding cellular heterogeneity. The effectiveness of clustering results and differential expression analysis heavily depends on the chosen data preprocessing workflows. Therefore, selecting appropriate analysis methods tailored to specific tissue types and dataset characteristics is particularly important for accurately identifying cell type classifications.
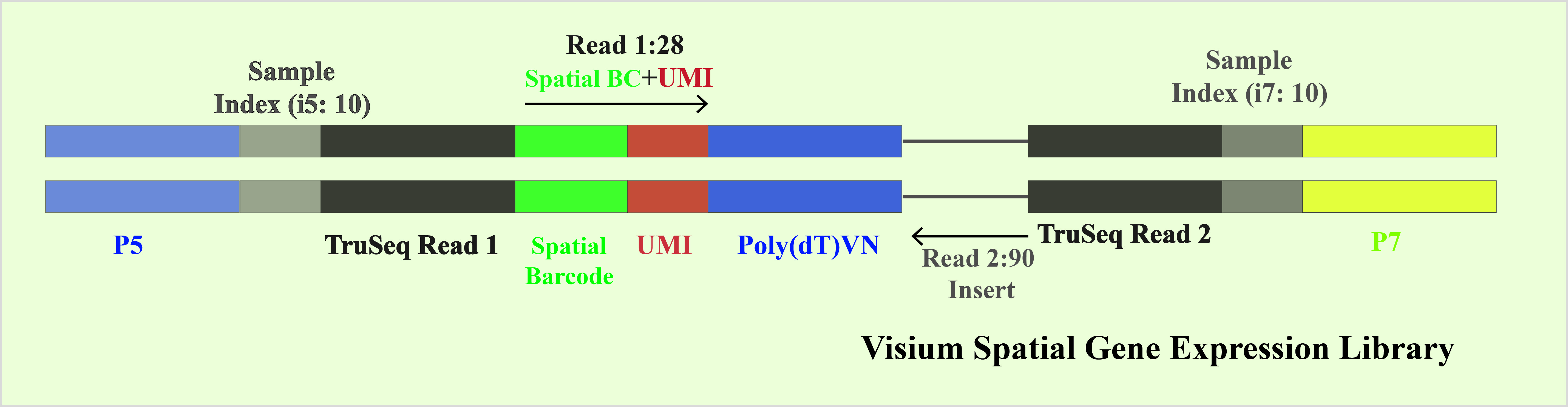
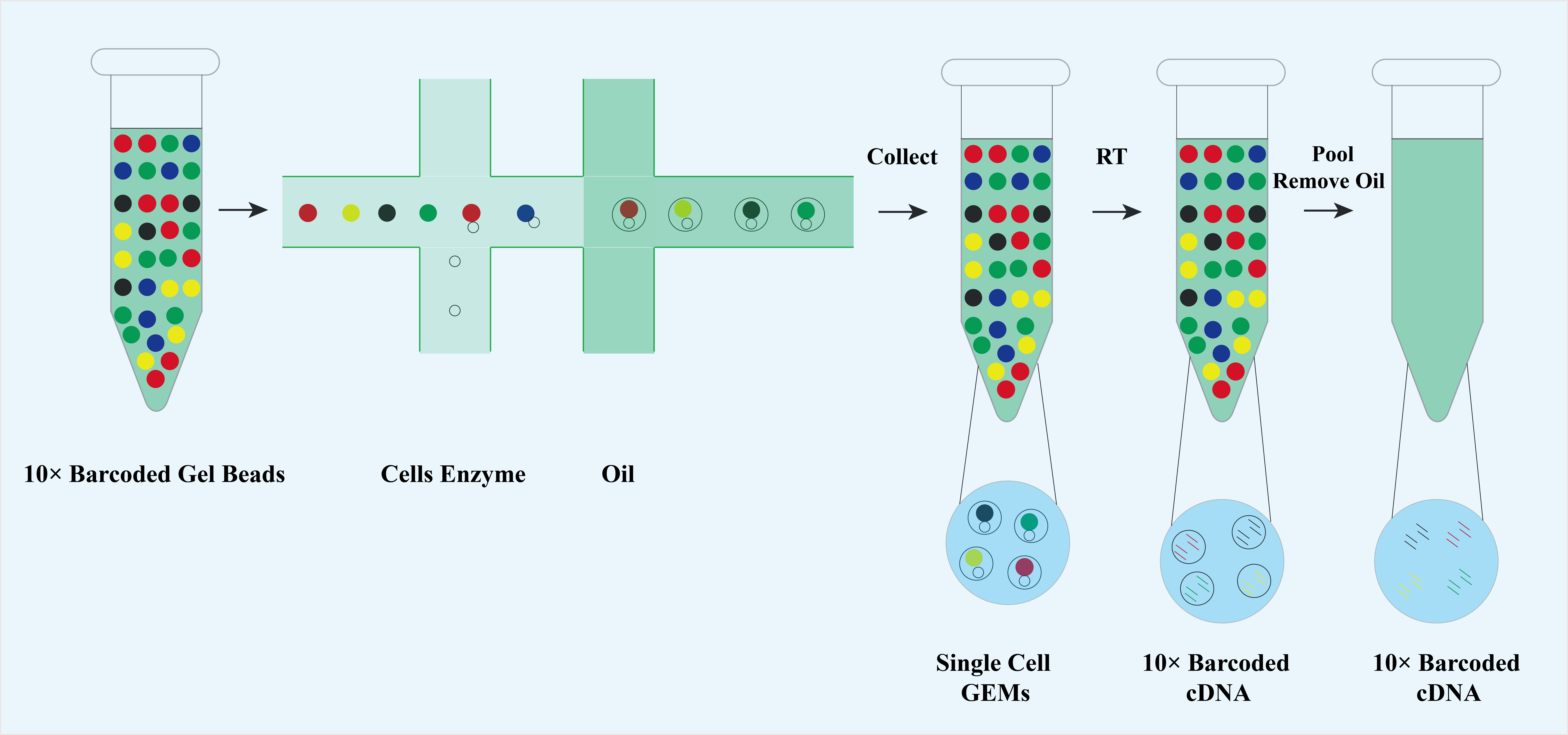
Cells are the most fundamental units within the tumor microenvironment, and tumor evolution exhibits significant heterogeneity between tumors and within tumors. In addition to tumor cells, various other cell types, including stromal cells (such as fibroblasts and endothelial cells) and immune cells (such as lymphocytes and monocytes-macrophages), also infiltrate the tumor, contributing to the heterogeneity of the tumor composition.
Inflammation-cancer transition is considered an important pathological process driving the occurrence of HCC, in which T cells and myeloid cells play the most significant roles. Zheng et al. [15] first revealed the immune status of T cells in HCC by employing scRNA-seq and T cell receptor (TCR) sequencing on 5,063 T cells from the peripheral blood, tumor, and adjacent tissues of six HCC patients. This analysis identified 11 T cell subpopulations and their developmental trajectories, highlighting an accumulation of regulatory T cells (Tregs) and exhausted T cells, which highly express LYNA, inhibiting CD8+ T cell function. Afterwards, the research team conducted a scRNA-seq study [16] using Smart-seq2 and 10x technologies on CD45+ immune cells from five tissue sites (primary tumor, adjacent tissue, lymph nodes, peripheral blood, and ascites) of 16 untreated HCC patients. The findings revealed that LAMP3+ dendritic cells (DCs), a subset of conventional DC subpopulations, migrated from the tumor to lymph nodes, expressing multiple immune ligand signals to interact with various immune cell subpopulations. Song et al. [17] analyzed tumors and adjacent tissues from seven HBV/HCV-related HCC cases and identified a new subset of activated T cells - XCL1+CD8+ T cells, associated with better overall survival. This analysis also identified a new type of tumor-associated macrophage (TAM) - CCL18+ TAM, characterized by high expression of CCL18 and the transcription factor CREM, indicative of M2 macrophage phenotype associated with poor prognosis and immune suppression. Previous studies have also reported the dysfunction of non-traditional T cells [18], such as γδ T cells and mucosal-associated invariant T cells (MAIT), in HCC.
Besides the well-studied immune cells, scRNA-seq technology has identified various stromal cells unique to the HCC microenvironment. Sun et al. [19] provided a comprehensive single-cell atlas, including fetal liver, HCC, and normal liver tissues. The findings identified embryonic-related endothelial cells and embryonic-like tumor-associated macrophages (FOLR2+ TAMs), exhibiting cross-species similarities. Spatial transcriptomics further revealed the presence of an immune-suppressive cancer-fetal ecosystem in HCC, characterized by PLVAP+ ECs, FOLR2+ TAMs, and Treg cells. Subsequent research elucidated this ecosystem [20]. Integrated spatial genomics revealed that POSTN+ cancer-associated fibroblasts (CAFs) spatially co-localize with PLVAP+ endothelial cells (ECs) and FOLR2+ tumor-associated macrophages (TAMs), forming interactions. Further analysis revealed associations between tumor embryonic reprogramming and epithelial-mesenchymal transition, tumor cell proliferation, and the recruitment of Treg cells. The TME in HCC is complex. Advanced scRNA-seq technology has uncovered a variety of previously unknown cell types unique to HCC. These cells exist exclusively within this environment and engage in intricate interactions with adjacent cells, creating a niche and enhancing the heterogeneity of HCC.
Study the mechanisms of HCC development and progression
scRNA-seq technology facilitates tracing and analyzing early cellular events and key factors driving HCC development. Zong et al. [21] discovered that AIF1+CSF1R+ mesenchymal stem cells appeared in the TME prior to the occurrence of HCC in a DEN-induced liver injury carcinogenesis model. Mechanistically, this activation might be mediated by TNF-α through the TNFR1/SIRT pathway, further promoting the recruitment of macrophages via the secretion of CCL5 and facilitating the occurrence of HCC. Immunoediting occurs throught the stages of HCC, yet changes in the TME from early to advanced stages remain poorly understood. Nguyen et al. [22] reported the gradual decrease in the number of antitumor immune subsets during the progression of HCC, while the exhausted subsets accumulate gradually. The abundance of CD8+ T cells peaks in tumors at TNM stage II, with significant alteration in the genes associated with antigen presentation and immune response, underscoring tumor-immune system co-evolution during HCC progression. Tsukamoto et al. [23] combined genetic lineage tracing and scRNA-seq to analyze the origin and molecular characteristics of PROM1+ cells, revealing important subpopulations in a mouse HCC model, including Prom1+Afp- ductal response cells and Prom1+Afp+ tumor-initiating cells.
The high recurrence rate of HCC hinders the improvement of overall survival, with postoperative 5-year recurrence rates as high as 50-70% [24]. Sun et al. [19] compared scRNA-seq datasets from 12 primary HCC and 6 recurrent HCC patients and found that in early recurrent tumors, the level of Tregs is reduced, while DCs and CD8+ T cells are increased, differing from the exhaustion state observed in primary tumors. The CD8+ T cells in recurrent tumors exhibit high expression of KLRB1 (which encodes the CD161 protein), indicating an inherently low cytotoxic state and clonal expansion. Differential gene expression and cell interactions revealed increased expression of immune checkpoint molecules (such as PD-L1) on HCC cells in recurrent lesions, which competes with CD80 on DCs, reducing CD80-CD28 binding and thereby inhibiting DC antigen presentation and preventing T cell activation. This study provides the first insights into the microenvironmental features of HCC recurrence, offering valuable information on immune escape mechanisms linked to tumor recurrence. Due to the potential differences in genomic mutation states and evolutionary trajectories between "true HCC recurrences" and "new lesions originating from different sources than the primary tumor," distinguishing between different recurrences could lead to developing specific therapeutic strategies tailored to each recurrence type.
Evolutionary trajectories and genomic heterogeneity often vary between true and de novo recurrences [25, 26], highlighting the importance of differentiating recurrence types based on biological characteristics to develop tailored treatment strategies. Chen et al. [27] utilized whole-exome sequencing to analyze the mutation status, evolutionary trajectories, and clonal architecture of recurrent HCC samples, identifying genuine recurrences from de novo lesions. Subsequently, 5' end scRNA-seq sequencing and V(D)J sequencing revealed an increased abundance of KLRB1+CD8+ T cells showing memory phenotypes and low cytotoxicity in genuine recurrences. In contrast, there were more cytotoxic T cells and exhausted CD8+ T cells in de novo lesions. Cell-cell communication analyses demonstrated that GDF15 expression increased in HCC cells adjacent to dendritic cells (DCs), possibly suppressing antigen presentation in genuine recurrences. At the same time, myeloid-cell and T-cell-mediated immune-suppressive signals were stronger in de novo lesions. In addition, the study reviewed Phase II clinical trials of neoadjuvant anti-PD-1 immunotherapy, which demonstrated better efficacy in patients with de novo HCC lesions, providing a new approach to guide recurrence HCC treatment by integrating genomic and scRNA-seq data.
Identifying new therapeutic targets and resistance mechanisms in HCC
Tumor cell heterogeneity promotes rapid adaptation to external pressures by recruiting and "conditioning" immune cells, thereby facilitating tumor survival and progression. Previous literature reports [28, 29] indicate that Sonic Hedgehog (SHH) secreted by HCC cells drives tumor-associated macrophages (TAMs) to polarize into the M2 state, reducing the expression of CXCL9/10 and thereby inhibiting the recruitment of CD8+ T cells to the tumor microenvironment (TME). Neutralizing SHH can reverse this immune suppression. Additionally, blocking the CCL2/CCR2 signaling axis, which inhibits the recruitment of inflammatory monocytes, TAM infiltration, and M2 polarization, can reverse the immune suppression and reactivate CD8+ T cell immune responses. Hao et al. [30] found that APOC1 is highly expressed on TAMs in HCC, significantly higher than in normal tissues, and is negatively correlated with the expression of PD1/PD-L1 in human HCC samples. Compared to wild-type mice, APOC1-/- C57BL/6J mice show a decreased proportion of M2 macrophages and increased levels of CD8+ T cells, M1 macrophages, and NK cells. The study suggests that targeting APOC1 can convert the M2 phenotype to the M1 phenotype by inhibiting the ferroptosis pathway in TAMs, thereby remodeling the tumor immune microenvironment and improving the efficacy of anti-PD-1 immunotherapy, providing a new strategy to enhance the effectiveness of anti-PD-1 treatment.
Circulating tumor cells (CTCs) provide the "seeds" for hematogenous dissemination in HCC and play a crucial role in tumor metastasis and drug resistance [31]. Sun et al. [32] analyzed single-cell sequencing data from 113 CTCs collected from four different vascular sites and found that the CTC transcriptome is closely related to stress responses, cell cycle, and immune evasion signals in blood circulation, with CCL5 identified as a key mediator of CTC immune evasion. Mechanistically, high expression of CCL5 in CTCs is regulated by the p38-MAX signaling pathway, which further recruits Tregs to promote metastasis. Therefore, targeting CCL5 or CCR5 could be a novel therapeutic approach for combating HCC metastasis.
The use of immune checkpoint inhibitors has become a first-line treatment for advanced HCC patients [33]. However, how different cell subpopulations in the tumor microenvironment (TME) mediate resistance to immune therapies such as anti-PD-1 remains poorly understood. Meng et al. [34] conducted scRNA-seq on 7 HCC patients undergoing anti-PD-1 immunotherapy (divided into sensitive and resistant groups) and discovered that NAMPT secreted by tumor cells reprograms CD10+ALPL+ neutrophils through NTRK1, maintaining them in an immature state. This process triggers a patient-specific irreversible exhausted T cell state in PD-1-resistant individuals. Zhou et al. [35] analyzed HCC patients receiving a combination of the anti-angiogenic drug Lenvatinib and anti-PD-1 therapy. They found that this combination led to an increase in the TNF-NFκB pathway across all immune cell subpopulations. Specifically, MAIT cells secreting TNF activate TNFRSF1B on Tregs, promoting immune suppression. Additionally, TNFSF9 was highly expressed on antitumor CD8+ T cells in the treatment group. This research provides further insights into immune therapy targets and the synergistic effects of combining anti-PD-1 treatments.
Promoting individualized treatment and prognostic evaluation of HCC
Using scRNA-seq technology has made significant progress in advancing personalized treatment and prognostic assessment of HCC. It has revealed the complex molecular landscape of HCC, making molecular subtyping, identification of prognostic biomarkers, and prediction of treatment outcomes more precise. By analyzing the expression profiles of individual cells, scRNA-seq helps identify different molecular subtypes within HCC, enabling classification based on these molecular features and facilitating the development of targeted therapeutic strategies. Cao et al. [36] identified marker genes for neutrophils from scRNA-seq data and integrated 101 machine-learning algorithms to stratify HCC patients based on neutrophil-related features. They assessed the clinicopathological characteristics and prognostic outcomes of HCC patients under different risk stratifications and validated their findings across multiple cohorts and omics levels. Additionally, they selected RTN3 from 10 hub genes for preliminary experimental validation, demonstrating high translational potential. Cai et al. [37] integrated public datasets to classify HCC patients into three subtypes with distinct metabolic characteristics: Type I, characterized by extracellular matrix production and upregulated glucose metabolism; Type II, which has some pathway abnormalities; and Type III, which features upregulated lipid metabolism. These subtypes exhibit different prognostic characteristics. Specifically, they found that ACADL, a mechanistic enzyme, can reprogram HCC metabolism by modulating extracellular matrix stiffness, making it a potential target for HCC treatment.
scRNA-seq also plays a crucial role in predicting HCC patients' responses to specific treatments. By providing a detailed analysis of the tumor microenvironment, including intercellular communication and identifying transcription factors that regulate gene expression, it is possible to infer the effects of certain therapeutic interventions. Roehlen et al. [38] utilized patient-derived in vitro and in vivo models along with highly specific CLDN1 monoclonal antibodies, combining traditional biological methods with scRNA-seq technology. They elucidated the mechanism by which CLDN1 monoclonal antibodies inhibit HCC progression. This inhibition occurs through suppressing hypoxia, tumor cell stemness, and reprogramming tumor metabolism. Specifically, the antibodies block the direct interaction between CLDN1 and JAG1, inhibiting downstream Notch signaling, affecting HCC progression. Liu et al. [39] used scRNA-seq combined with spatial transcriptomics to identify structural differences and cell subpopulation distributions in the tumor microenvironment of HCC patients who responded to and did not respond to immunotherapy. They revealed a tumor immune barrier composed of SPP1+ tumor-associated macrophages (TAM) and cancer-associated fibroblasts (CAF). By analyzing the ligand-receptor network between tumor cells, SPP1+ TAM, and CAF, they demonstrated the significant role of hypoxic signals in the formation of SPP1+ TAM, which further stimulated CAF to secrete extracellular matrix, thereby limiting immune infiltration within the tumor.
In the entire process of scRNA-seq, various challenges are involved, ranging from sample preparation to data analysis, with room for improvement at each step. Issues such as cell damage, cell viability, factors affecting reverse transcription efficiency, and contamination by free RNA need to be addressed to obtain high-quality GEMs (Gel Bead-in-Emulsions) from solid tumors or other tissues. Due to the large and complex data generated from sequencing each cell in scRNA-seq, computational performance challenges are present when handling large-scale datasets. Additionally, the comprehensiveness of scRNA-seq is often accompanied by insufficient sequencing depth. Balancing resolution with sensitivity remains a limiting factor in technological development. Future advancements need to focus on improving the detection of low-abundance transcripts. Another challenge is distinguishing between true biological variability and technical noise in scRNA-seq data. Due to the data sparsity and high dropout rates caused by the sequencing methods, complex computational strategies are required to interpret the results accurately. Accurately identifying and annotating different cell types and states is a significant challenge, especially without clear subpopulation markers.
Challenges in clinical applications
The development of scRNA-seq technology provides a new perspective, allowing us to understand the dynamic changes in biological systems with higher resolution and track cell fate. The potential applications of this technology extend beyond basic research in the laboratory to the clinical field, offering new possibilities for clinical diagnosis and treatment. However, translating scRNA-seq technology from the laboratory to routine clinical practice faces several challenges. One of the main challenges is cost-effectiveness. Although sequencing costs have gradually decreased with advances in sequencing technology and computational tools, standardization and validation consistency costs remain significant and cannot be overlooked. Therefore, to achieve widespread clinical use of scRNA-seq, it is crucial to address the challenge of rapidly and accurately interpreting large volumes of data and integrating this information with the patient's disease background to provide appropriate treatment recommendations. In addition to cost issues, several other factors need to be considered. When conducting research with potential clinical applications, it is essential to protect patient privacy and data, adhere to ethical guidelines, and follow legal regulations. Resolving these issues is critical for the sustainable development of the technology and the success of its clinical applications and warrants thorough exploration in future research and practice.
Future direction
With the continuous development and advancement of scRNA-seq, it has become one of the key technologies in the biomedical field. In the future, the development of this technology may focus on several key directions: (1) Technological innovation: Continuously improving current sequencing methods and developing new tools, including further enhancing sequencing resolution and developing new algorithms and tools. (2) Integration of multimodal single-cell sequencing data: Integrating single-cell multi-omics technologies such as genomics, transcriptomics, epigenomics, and proteomics around central principles to provide a more comprehensive understanding of cell function and status in major diseases like HCC. (3) Clinical diagnosis and treatment: In the future, scRNA-seq technology holds promising potential for precision medicine. There are reports on the practical applications of single-cell technologies in identifying early disease markers, tracking tumor evolution, and revealing clonal evolution in diseases such as AML (acute myeloid leukemia) and chronic lymphocytic leukemia [40]. (4) Artificial intelligence and machine learning: The large datasets generated by scRNA-seq can leverage artificial intelligence and machine learning to improve data analysis efficiency. This synergy fosters the development of new algorithms and tools, further optimizing data analysis models and creating a positive feedback loop to provide deeper biological insights. (5) Collaboration and data sharing: International collaboration and data-sharing platforms are becoming increasingly important in advancing disease research. Shared databases and collaborative research help aggregate and analyze data from various sources, deepening understanding of complex diseases and driving medical advancements.
In summary, this article thoroughly explores the progress of scRNA-seq technology in HCC research, highlighting the challenges and future directions. It aims to equip researchers with a comprehensive perspective of the current status and future potential of scRNA-seq. This research is vital for developing novel treatment strategies and enhancing treatment outcomes by analyzing the heterogeneity of HCC and providing insights at subpopulation and cellular levels. Furthermore, it will significantly bolster efforts toward achieving precision medicine. The technological advancements and increasing interdisciplinary collaboration have ample reason to anticipate that single-cell technologies will play a significant role in uncovering the biological essence of complex diseases like HCC and advancing personalized healthcare.
No applicable.
Availability of data and materials
Data and materials are available on request from the authors.
Ethical policy
Not applicable.
Author contributions
SN conceptualized, designed, conducted research, wrote the draft and approve the final manuscript.
Competing interests
The authors have no conflicts of interest regarding the publication of this paper.
Funding
None.
- Siegel R L, Miller K D, Wagle N S, Jemal A D: Cancer Statistics, 2023. CA Cancer J Clin 2023, 73(1): 17- 48.
- Liu Z, Lin C, Mao X, Guo C, Suo C, Zhu D, Jiang W, Li Y, Fan J, Song C, et al: Changing Prevalence of Chronic Hepatitis B Virus Infection in China between 1973 and 2021: A Systematic Literature Review and Meta-Analysis of 3740 Studies and 231 Million People. Gut 2023, 72(12): 2354-2363.
- Yang J D, Heimbach J K: New Advances in the Diagnosis and Management of Hepatocellular Carcinoma. BMJ 2020, 371: m3544.
- Vogel A, Meyer T, Sapisochin G, Salem R, Saborowski A: Hepatocellular Carcinoma. Lancet 2022, 400(10360): 1345-1362.
- Huang A, Yang X R, Chung W Y, Dennison A R, Zhou J: Targeted Therapy for Hepatocellular Carcinoma. Signal Transduct Target Ther 2020, 5(1): 146-159.
- Sangro B, Sarobe P, Hervas-Stubbs S, Melero I: Advances in Immunotherapy for Hepatocellular Carcinoma. Nat Rev Gastroenterol Hepatol 2021, 18(8): 525-543.
- Chan L K, Tsui Y M, Ho D W, Ng I O: Cellular Heterogeneity and Plasticity in Liver Cancer. Semin Cancer Biol 2022, 82(10): 134-149.
- Sul J Y, Wu C W, Zeng F, Jochems J, Lee M T, Kim T K, Peritz T, Buckley P, Cappelleri D J, Maronski M, et al: Transcriptome Transfer Produces a Predictable Cellular Phenotype. Proc Natl Acad Sci USA 2009, 106(18): 7624-7629.
- Elmentaite R, Dominguez Conde C, Yang L, Teichmann S A: Single-Cell Atlases: Shared and Tissue Specific Cell Types across Human Organs. Nat Rev Genet 2022, 23(7): 395-410.
- Gross A, Schoendube J, Zimmermann S, Steeb M, Zengerle R, Koltay P: Technologies for Single-Cell Isolation. Int J Mol Sci 2015, 16(8): 16897-16919.
- Jovic D, Liang X, Zeng H, Lin L, Xu F, Luo Y: Single-Cell Rna Sequencing Technologies and Applications: A Brief Overview: Clin Transl Med 2022, 12(3): e694.
- Van Galen P, Hovestadt, V, Wadsworth I, Marc H, Hughes T K, Griffin G K, Battaglia S, Verga J A, Stephansky J, Pastika T J, et al: Single-Cell RNA-Seq Reveals AML Hierarchies Relevant to Disease Progression and Immunity. Cell 2019, 176(6): 1265-1281.
- Hao Y, Stuart T, Kowalski M H, Choudhary S, Hoffman P, Hartman A, Srivastava A, Molla G, Madad S, Fernandez-Granda C, et al: Dictionary Learning for Integrative, Multimodal and Scalable Single-Cell Analysis. Nat Biotechnol 2024, 42(2): 293-304.
- Korsunsky I, Millard N, Fan J, Slowikowski K, Zhang F, Wei K, Baglaenko Y, Brenner M, Loh P R, Raychaudhuri S: Fast, Sensitive and Accurate Integration of Single-Cell Data with Harmony. Nat Methods 2019, 16(12): 1289-1296.
- Zheng C, Zheng L, Yoo J K, Guo H, Zhang Y, Guo X, Kang B, Hu R, Huang J Y, Zhang Q, et al: Landscape of Infiltrating T Cells in Liver Cancer Revealed by SingleCell Sequencing. Cell 2017, 169(7): 1342-1356.
- Zhang Q, He Y, Luo N, Patel S J, Han Y, Gao R, Modak M, Carotta S, Haslinger C, Kind D, Peet G W, et al: Landscape and Dynamics of Single Immune Cells in Hepatocellular Carcinoma. Cell 2019, 179(4): 829-845.
- Song G, Shi Y, Zhang M, Goswami S, Afridi S, Meng L, Ma J, Chen Y, Lin Y, Zhang J, et al: Global Immune Characterization of Hbv/Hcv-Related Hepatocellular Carcinoma Identifies Macrophage and T-Cell Subsets Associated with Disease Progression. Cell Discov 2020, 6(1): 90-108.
- Yao T, Shooshtari P, Haeryfar S M M: Leveraging Public Single-Cell and Bulk Transcriptomic Datasets to Delineate Mait Cell Roles and Phenotypic Characteristics in Human Malignancies. Front Immunol 2020, 11: 1691.
- Sun Y, Wu L, Zhong Y, Zhou K, Hou Y, Wang Z, Zhang Z, Xie J, Wang C, Chen D, et al: Single-Cell Landscape of the Ecosystem in Early-Relapse Hepatocellular Carcinoma. Cell 2021, 184(2): 404-421.
- Li Z, Pai R, Gupta S, Currenti J, Guo W, Di Bartolomeo A, Feng H, Zhang Z, Li Z, Liu L, et al: Presence of Onco-Fetal Neighborhoods in Hepatocellular Carcinoma Is Associated with Relapse and Response to Immunotherapy. Nat Cancer 2024, 5(1): 167-186.
- Zong C, Meng Y, Ye F, Yang X, Li R, Jiang J, Zhao Q, Gao L, Han Z, Wei L: Aif1+ Csf1r+ Mscs, Induced by Tnf-Alpha, Act to Generate an Inflammatory Microenvironment and Promote Hepatocarcinogenesis. Hepatology 2023, 78(2): 434-451.
- Nguyen PHD, Wasser M, Tan CT, Lim CJ, Lai HLH, Seow JJW, Dasgupta R, Phua CZJ, Ma S, Yang J, et al: Trajectory of Immune Evasion and Cancer Progression in Hepatocellular Carcinoma. Nat Commun 2022, 13(1): 1441-1460.
- Wu R, Pan S, Chen Y, Nakano Y, Li M, Balog S, Tsukamoto H: Fate and Functional Roles of Prominin 1(+) Cells in Liver Injury and Cancer. Sci Rep 2020, 10(1): 19412-19428.
- European Association for the Study of the Liver. Electronic Address E E E, European Association for the Study of The L: Easl Clinical Practice Guidelines: Management of Hepatocellular Carcinoma. J Hepatol 2018, 69(1): 182-236.
- Ding X, He M, Chan AWH, Song QX, Sze SC, Chen H, Man M KH, Man K, Chan SL, Lai PBS, et al: Genomic and Epigenomic Features of Primary and Recurrent Hepatocellular Carcinomas. Gastroenterology 2019, 157(6): 1630-1645.
- Chen G, Cai Z, Li Z, Dong X, Xu H, Lin J, Chen L, Zhang H, Liu X, Liu J: Clonal Evolution in LongTerm Follow-up Patients with Hepatocellular Carcinoma. Int J Cancer 2018, 143(11): 2862-2870.
- Chen S, Huang C, Liao G, Sun H, Xie Y, Liao C, Wang J, He M, Hu H, Dai Z, et al: Distinct Single-Cell Immune Ecosystems Distinguish True and De Novo Hbv-Related Hepatocellular Carcinoma Recurrences. Gut 2023, 72(6): 1196-1210.
- Li X, Yao W, Yuan Y, Chen P, Li B, Li J, Chu R, Song H, Xie D, Jiang X, et al: Targeting of TumourInfiltrating Macrophages Via Ccl2/Ccr2 Signalling as a Therapeutic Strategy against Hepatocellular Carcinoma. Gut 2017, 66(1): 157-167.
- Petty A J, Li A, Wang X, Dai R, Heyman B, Hsu D, Huang X, Yang Y: Hedgehog Signaling Promotes Tumor-Associated Macrophage Polarization to Suppress Intratumoral CD8+ T Cell Recruitment. J Clin Invest 2019, 129(12): 5151-5162.
- Hao X, Zheng Z, Liu H, Zhang Y, Kang J, Kong X, Rong D, Sun G, Sun G, Liu L, et al: Inhibition of Apoc1 Promotes the Transformation of M2 into M1 Macrophages Via the Ferroptosis Pathway and Enhances Anti-Pd1 Immunotherapy in Hepatocellular Carcinoma Based on Single-Cell Rna Sequencing. Redox Biol 2022, 56: 102463.
- Ogle LF, Orr JG, Willoughby CE, Hutton C, Mcpherson S, Plummer R, Boddy AV, Curtin NJ, Jamieson D, Reeves HL: Imagestream Detection and Characterisation of Circulating Tumour Cells - a Liquid Biopsy for Hepatocellular Carcinoma? J Hepatol 2016, 65(2): 305-313.
- Sun YF, Wu L, Liu SP, Jiang MM, Hu B, Zhou KQ, Guo W, Xu Y, Zhong Y, Zhou XR, et al: Dissecting Spatial Heterogeneity and the Immune-Evasion Mechanism of Ctcs by Single-Cell Rna-Seq in Hepatocellular Carcinoma. Nat Commun 2021, 12(1): 4091-4101.
- Feng MY, Chan LL, Chan SL: Drug Treatment for Advanced Hepatocellular Carcinoma: First-Line and Beyond. Curr Oncol 2022, 29(8): 5489-5507.
- Meng Y, Ye F, Nie P, Zhao Q, An L, Wang W, Qu S, Shen Z, Cao Z, Zhang X, et al: Immunosuppressive Cd10(+)Alpl(+) Neutrophils Promote Resistance to Anti-Pd-1 Therapy in Hcc by Mediating Irreversible Exhaustion of T Cells. J Hepatol 2023, 79(6): 1435-1449.
- Zhou C, Sun BY, Zhou PY, Yang ZF, Wang ZT, Liu G, Gan W, Wang Z, Zhou J, Fan J, et al: Mait Cells Confer Resistance to Lenvatinib Plus Anti-Pd1 Antibodies in Hepatocellular Carcinoma through Tnf-Tnfrsf1b Pathway. Clin Immunol 2023, 256: 109770.
- Gong Q, Chen X, Liu F, Cao Y: Machine Learning-Based Integration Develops a Neutrophil-Derived Signature for Improving Outcomes in Hepatocellular Carcinoma. Front Immunol 2023, 14: 1216585.
- Cai J, Chen T, Jiang Z, Yan J, Ye Z, Ruan Y, Tao L, Shen Z, Liang X, Wang Y, et al: Bulk and single-cell transcriptome profiling reveal extracellular matrix mechanical regulation of lipid metabolism reprograming through YAP/TEAD4/ACADL axis in hepatocellular carcinoma. Int J Biol Sci 2023, 19(7): 2114-2131.
- Roehlen N, Muller M, Nehme Z, Crouchet E, Juhling F, Del Zompo F, Cherradi S, Duong FHT, Almeida N, Saviano A, et al: Treatment of Hcc with Claudin-1-Specific Antibodies Suppresses Carcinogenic Signaling and Reprograms the Tumor Microenvironment. J Hepatol 2023, 78(2): 343-355.
- Liu Y, Xun Z, Ma K, Liang S, Li X, Zhou S, Sun L, Liu Y, Du Y, Guo X, et al: Identification of a Tumour Immune Barrier in the Hcc Microenvironment That Determines the Efficacy of Immunotherapy. J Hepatol 2023, 78(4): 770-782.
- Lim J, Chin V, Fairfax K, Moutinho C, Suan D, Ji H, Powell JE: Transitioning Single-Cell Genomics into the Clinic. Nat Rev Genet 2023, 24(8): 573-584.
Asia-Pacific Journal of Oncology
print ISSN: 2708-7980, online ISSN: 2708-7999
Copyright © Asia Pac J Oncol. This work is licensed under a Creative Commons Attribution-NonCommercial-No Derivatives 4.0 International (CC BY-NC-ND 4.0) License.